What is data quality?
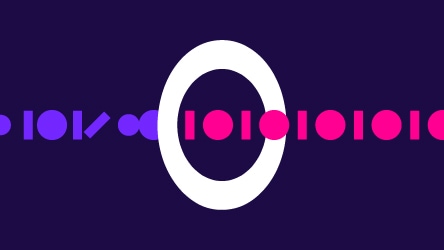
An ultimate guide to what is data quality and why is it important
This ultimate guide dives into the question, “What is data quality, and why is it important?” Our data quality experts will explain the importance of data quality, the benefits it offers businesses and people, how to implement data quality management into your organization, and much more.
It is tempting to believe that data and the management of its quality is something new, brought about by the advent of new regulations such as E-Privacy and the EU GDPR. It is not. Data, its management, and its quality have been around since information was first created: when we started writing things down.
What is data quality? | Data quality definition
We could go further, talking about what is data quality as a process, making data operational, enabling individuals and organizations to draw insights from the data which will inform their decision-making.
The reason we describe data quality as a process rather than a single item is that it comprises various elements that all contribute to the purpose of making data “fit for purpose”. Sometimes people use the term Data Preparation to refer to these elements, though data prep should be considered separate for now.
Why is data quality important?
The importance of data quality lies in the value it offers to businesses.
Low-quality data can have a significant negative impact on businesses by increasing the risk of inaccurate and misinformed data, which ultimately leads to poor business strategies and decisions being made.
Our definition of data quality’s value is this: what are the business, risk, and financial values assigned to any piece of information?
In this manner, data analysts and other practitioners of data management can quickly assign priorities to different data sources or specific data domains when they do data quality projects.
We recommend using a tool to assign literal values to your data, here are some examples:
Business – How valuable is Employee salary data to marketing? Chances are, it has a much higher business value to the HR department, whereas customer emails are more useful for marketing.
Risk – Are you holding Personally Identifiable Information (PII)? This means you could be exposed to the risk of GDPR fines if this data is not accurately protected to ensure the individual’s privacy.
Financial – eCommerce companies are the best example of the financial value of data. Typically, email addresses and credit card numbers are all that is needed in order to transact with customers. Therefore, profiling the data, keeping it of high quality, and reporting it over time can help eCommerce businesses understand the average value of customers and accurate email addresses.
As you can see from these examples, the importance of data quality tools can quickly become mission-critical for your business, depending on the quality of the data you hold that you need to perform day-to-day operations.To summarize the question of “Why is data quality important?” Because trustworthy and accurate data support better decision-making that increases the longevity and profitability of companies.
What are the benefits of improved data quality?
There are so many benefits to improving the quality of your information, but the top takeaways for businesses are:
Benefit | Real‑world impact |
---|---|
Trustworthy analytics | Reports and dashboards that stakeholders actually use |
Marketing ROI | Better deliverability and targeting of email and post improve campaign return |
Cost and time savings | Fewer manual fixes and process errors save $1-10 per record. |
Personalization | Tailored offers that boost conversion |
Faster decisions | Improved, smarter decision-making |
Regulatory compliance | Avoid hefty fines by complying with new and existing regulations, create a consumer-centric, data-driven culture. |
Productivity | Data workers spend less time cleansing, more time innovating |
Competitive forecasting | Accurate models predicting future demand and opportunities that will give your company a competitive advantage. |
Ultimately, your business is unique and how you benefit from improved data quality will also be unique. The opportunities and benefits that better data quality can offer businesses are endless.
What are the dimensions of data quality?
The dimensions of data quality are made up of six elements: Completeness, Validity, Timeliness, Uniqueness, Accuracy, and Consistency. We’ll explain each of these in more detail below:
Six dimensions of data quality – quick overview
Dimension | What it answers | Example |
---|---|---|
Completeness | Are all required values present? | Phone & email both captured for billing |
Validity | Does each value follow the correct format or range? | Postal code matches country pattern |
Timeliness | Is the data available when needed? | Sales figures refreshed hourly |
Uniqueness | Are there duplicates? | One order ID = one record |
Accuracy | Does the data reflect reality? | GPS coordinates match store location |
Consistency | Does data agree across systems? | Same customer status in CRM & ERP |
1. Completeness
Completeness ensures that all the values and types are accounted for in the data set. Are there gaps in the data, and if so, where? Some gaps are worse than others and what is considered a gap depends on the process where the data is used. For example, if the billing department requires both a phone number and email address, then no record missing one or the other can be considered complete. You can also measure completeness for any particular column. Profiling your data will uncover these gaps.
2. Validity
As the second dimension of data quality, validity checks verify that the data conforms to a particular format, data type, and range of values.
Are the postcode records you hold in a valid format? How confident are you that the email and postal address records you hold in your database are capable of receiving? Validity checks verify that the conforms to a particular format, data type, and range of values.
Since data-driven automation is so important nowadays, data has to be valid to be accepted by processes and systems that expect it.
3. Timeliness
Timeliness is a crucial dimension because of the increasing need for up-to-date data. Is new information entering your CRM every day in real-time or are you manually importing it? How often is the data “refreshed”?
Similar to other dimensions, timeliness is user-defined. One kind of data needs to be available on a quarterly basis for financial reporting. Other data must not be older than 5 minutes for real-time analytics.
4. Uniqueness
Uniqueness measures how much duplicate data there is in a given data set, either within any particular column or as whole records.
Do you have the same customer recorded twice in your data set or data catalog? For example, in the orders table, each order should have just one row. If, on the other hand, you encounter two records with the same order ID, you have a duplicate. How did it get there? Someone could have mistyped the order number. This brings us to the next dimension: accuracy.
5. Accuracy
Perhaps the most important dimension of data quality, accuracy, refers to the number of errors in the data. In other words, it measures to what extent recorded data represents the truth. Accuracy is tricky because data might be valid, timely, unique, and complete, but inaccurate.
100% accuracy is an aspirational goal for many data managers, and once achieved, the principles of data governance can be combined with data quality to ensure the data does not degrade and become inaccurate ever again.
6. Consistency
Consistency ensures that the data is uniform across the board, reducing internal conflicts in data sets.
Do you have conflicting information about the same customer in two different systems? That means the data is inconsistent, which might lead to inconsistent reporting and poor customer service.
What are the business costs and risks of poor data quality?
Data quality maturity curves are becoming more prevalent, and organizations can quickly ascertain whether they’re reactive or optimized and governed in their approach to data management.
An example of an organization that is immature in its capture and management of data is one that does not use validation fields or uses free-form capture fields on the contact forms of its website, allowing anyone to enter whatever they like.
Bad data should not be taken lightly as it poses significant risks and business costs. Below are several examples:
- Wasted marketing budget: if your organization sends physical mail to your customers and marketing leads but those addresses are outdated or invalid, you’ll be wasting precious marketing dollars and time.
- Non-compliant data: Regulations, such as GDPR, require a certain standard (Article 5) of how to maintain Data Quality in relation to the accuracy and integrity of data. If an organization’s data is found to be non-compliant with data-driven regulations, such as the EU General Data Protection Regulation (GDPR), they can be fined up to 20 million euros or 4% of annual turnover – whatever is higher!
- Hindered IT modernization projects: when data moves from source to target system, without correct mapping and data quality tools, old dirty data can wreak havoc on the new system.
- Poor customer experience: If contact information is of poor quality, you cannot provide customers with a tailored customer experience and serve them via their preferred channel.
- Fines: In regulated industries such as healthcare and banking, enterprises risk miscalculating key statistics for regulatory reports and getting fined.
- Unreliable analytics and machine learning: Inaccurate or invalid data will provide inaccurate analytics and unreliable machine learning models.
- Strategic operational mistakes: Building a warehouse at the wrong location, not catching fraud, and producing the wrong alloy are all examples of using bad data for business decision-making.
And yes, you can put a number on data quality.
What are must-have features to ensure data quality?
Data Profiling
Before you do any data quality checks, it’s important to examine your data at its source to better interpret and understand it. Data profiling does this faster and more efficiently than via SQL queries. It helps with defining what transformations are necessary for the data and what problems to track in the future.
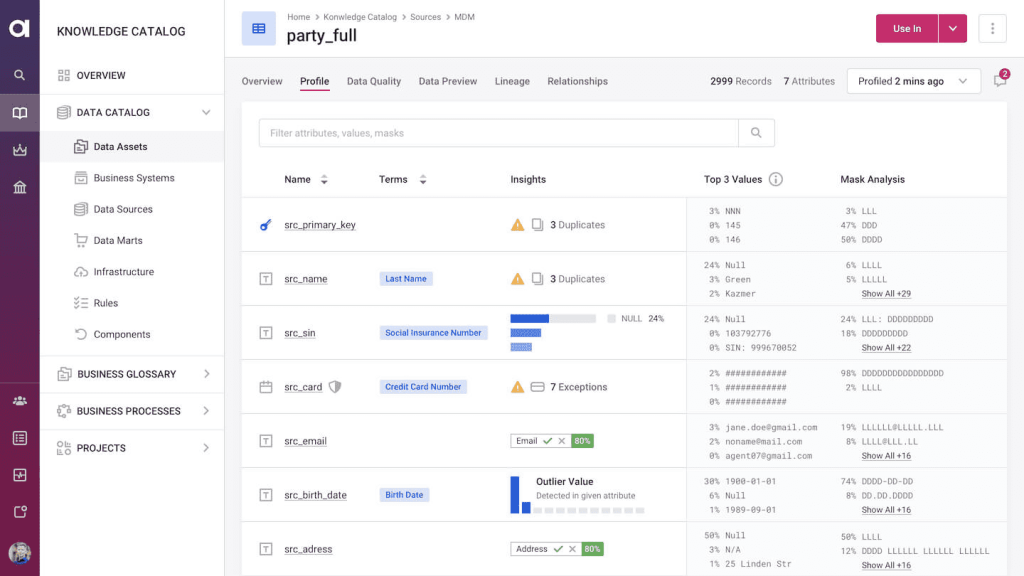
Data cleansing and transformation
Very often you need to transform data to improve its quality. This includes:
- Format standardization
- Parsing data and breaking it down into separate attributes (e.g., full name into first name and last name)
- Data enrichment: bringing additional data from external sources
- Data deduplication: remove duplicates from data
- Data masking: sometimes you need to obfuscate data for security reasons
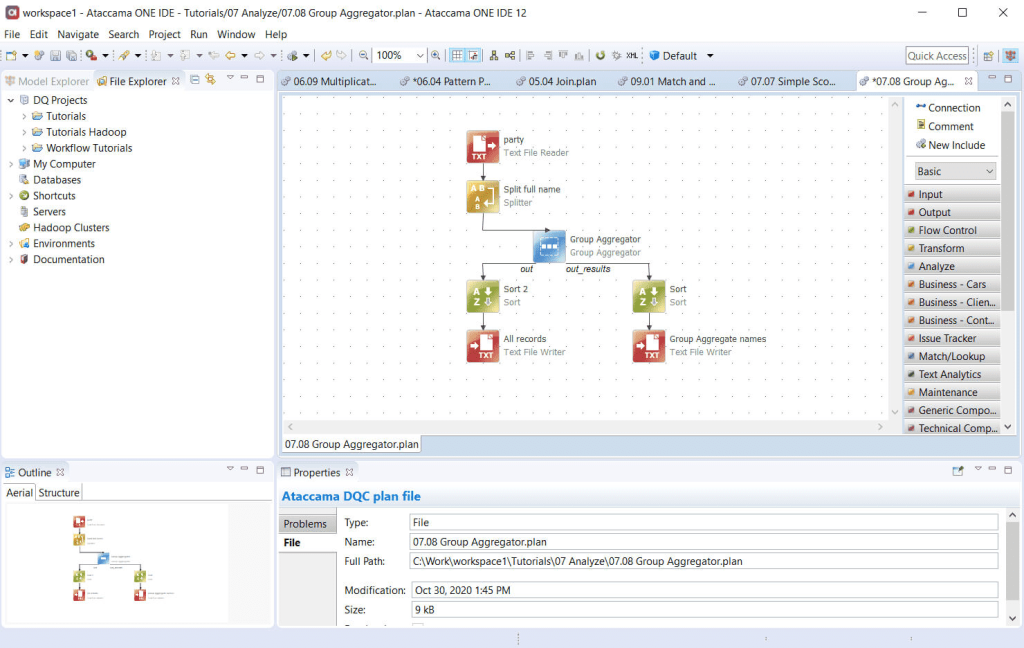
It’s important to note that these processes need to happen automatically to any new data before it travels to other systems and data analysts, and is used for business decision-making.
That being said, it’s even more beneficial and smart to establish processes that validate and “treat data” before it enters any IT system. This is called a data quality firewall. An example of this is an algorithm that checks data entered into a web form against a required format and alerts the user to fix it, such as email addresses or birth dates. However, data quality firewalls can be embedded into complex enterprise applications as well.
Monitoring and reporting
Peter Drucker said it best: “If you can’t measure it, you can’t improve it.” It’s as valid data quality as it is for business in general. Tracking changes and improvements to data over time is crucial and is usually done through data quality dashboards.
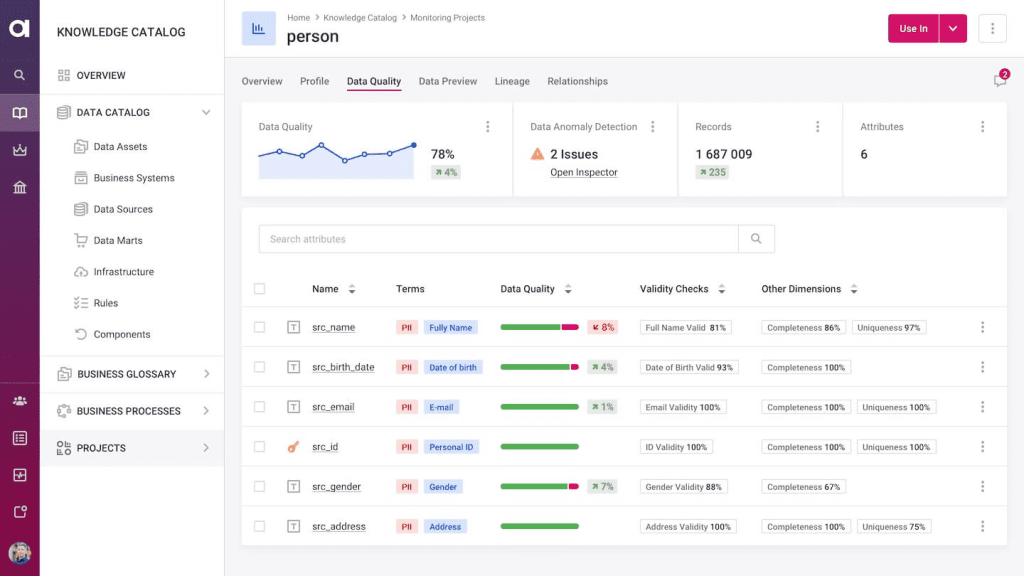
- First, it shows you whether you are moving in the right direction, i.e., whether the data quality metrics that you have defined are improving or not.
- Second, monitoring data quality helps catch unexpected influxes of bad data and track it to its source.
- And third, it helps with tracking compliance with regulatory requirements and more.
If you’d like to learn about all the essential capabilities of data quality, you can read the full article here.
Data quality FAQ
If you want to know more, here are some frequently asked questions about the importance of data quality.
1. What is data quality vs data integrity?
While the two are often used interchangeably, there is a clear difference between data quality and data integrity.
Data Quality: Focuses on six dimensions of data quality to ensure that the data is reliable, accurate, and valuable to the recipient. Those dimensions of data quality are:
- Completeness
- Validity
- Timeliness
- Uniqueness
- Accuracy
- Consistency
Data Integrity: Focuses mostly on the dependability and security of the data. The physical integrity of “data integrity” focuses on security measures and access controls to prevent data corruption of unauthorized parties.
2. Can the data catalog and data quality work together?
Yes! Monitoring your data quality is much more efficient and accessible when integrating it with your data catalog. More specifically, you can automate data quality workflows using the metadata from the data catalog. Here are other ways the data catalog and data quality benefit each other:
- Automating data quality monitoring
- Improving data discovery
- Streamlining on-demand DQ evaluation
- Simplifying data preparation
- Helping discover root causes of quality issues
3. What is a real-world example of bad data quality affecting analytics?
One of the most common places we find data quality is during census analysis. Many censuses are taken in paper and digital format, leading to quality discrepancies like unreadable inputs and duplicate entries for the same applicant. Most census data undergoes data profiling, standardization, enrichment, matching and consolidation, and relationship discovery before it’s considered fit for analysis.
4. How do I get started with data quality improvement and management?
Data quality management can seem like a bit of a daunting task. Follow the steps below on how to get started with data quality management.
- Determine your current goals and scope (help with a specific business problem dependent on data or focus on a specific critical data element).
- Profile your data.
- Fix the most urgent issues as soon as possible.
- Come up with metrics and methods for measuring its quality.
- Monitor data quality problems.
- Scale your program to other teams, departments, source systems, and critical data elements.
Following this process will ensure you find the relevant strategy for your organization and won’t embark on a task that is overwhelming or inadequate.
5. How important is data quality for successful AI implementations?
Data quality is essential for successful AI implementations. Spending too much time preparing data is one of the main reasons AI is so expensive and time-consuming. You can ensure more successful AI implementations if you:
- Profile your data
- Perform data quality evaluations
- Have regular data quality monitoring
Otherwise, you’ll be building machine learning models on the wrong sets, inevitably leading to errors or more work for your AI architects.
6. Where is Data Quality headed in the future?
Data quality is undoubtedly here to stay, but what kind of innovations can we expect? Well, you can expect the following improvements in the next few years:
- Further automation will enable greater adoption of new architectures like the data fabric and data mesh.
- The term is growing to encompass other aspects of data management like reference and master data management.
- Data being deliverable to any user at the company regardless of skillset.
- Data quality tools are becoming singular solutions instead of fragmented features that can cause conflict.
- More systems than people are consuming data.
- Much more!
Improve your data quality with Ataccama’s data quality management platform!
Invest in enhancing your business’ data with the experts in the industry! Ataccama’s sophisticated data quality software makes it easy for you to reduce costs, regain valuable time, and increase data quality and accessibility across the organization.
Get in touch with our team today for more information on how you can get started or schedule a demo to see the platform in action yourself!
Not ready to jump in quite yet? Learn how we’ve helped support enterprise organizations like T-Mobile and Raiffeisenbank. Check out our customer success stories!