3 Data quality management success factors
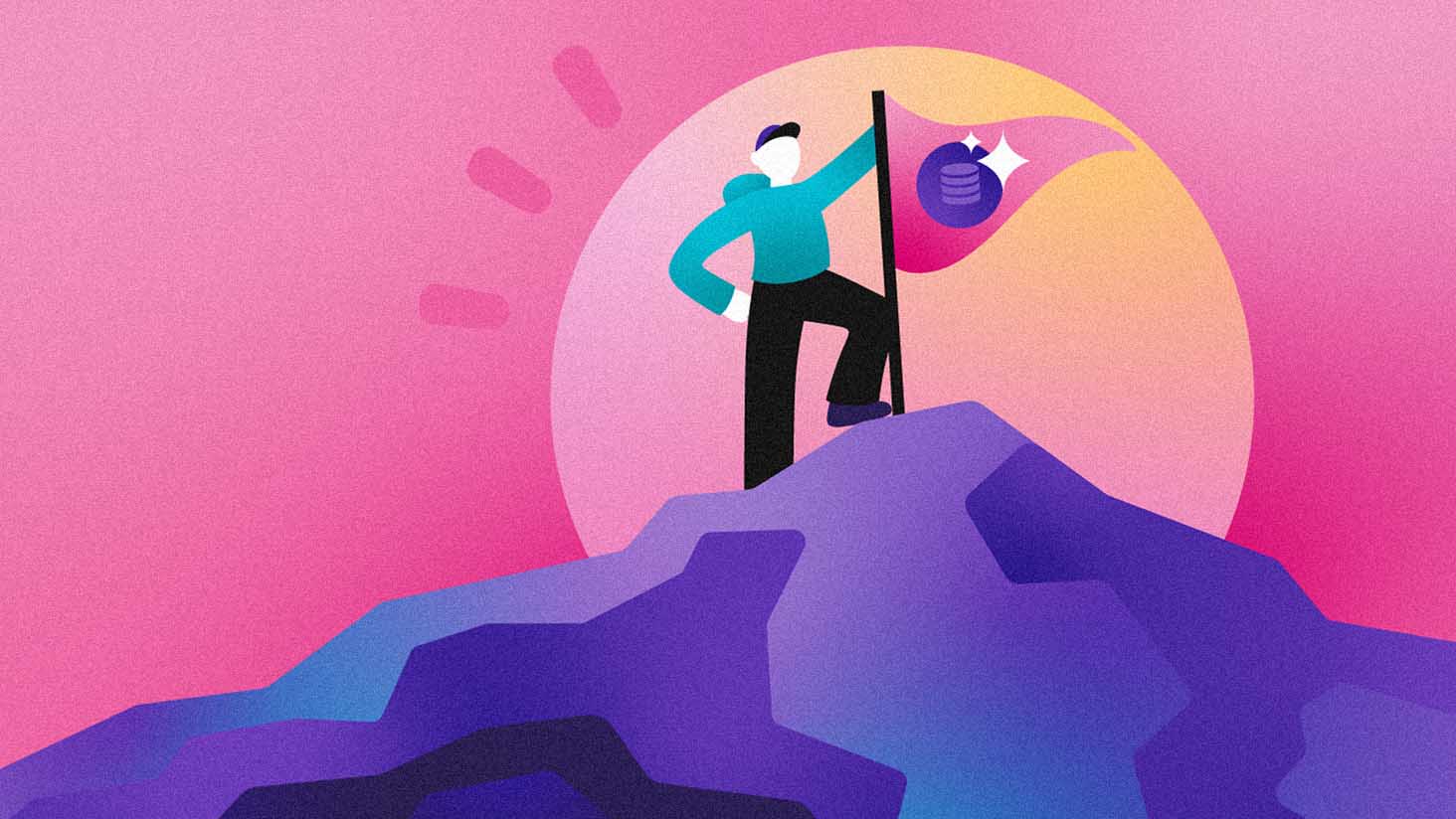
Every year we survey the data management market to stay up to date with the latest trends, pains, practices, and innovations.
The 2022 edition unveiled three factors that lead to data quality management success:
- Automation
- Infrastructure
- Standardized deployment & commitment to DQ practices
Continue reading to learn more!
Success factor #1: Automation
The number 1 factor correlated with success in data quality management is automation. As the authors of the 2022 State of Data Quality report wrote:
“DQM automation delivers results. Automation is one of the paramount success factors for DQM.”
Successful companies had, on average, 70% of their DQM processes automated, while the unsuccessful ones had only 19% automated. We also found that automation was much more common in larger organizations. None of this is a surprise because automation and AI support and provide what data people want: self-service and real-time capabilities. They are also, of course, substantial time savers.
But what role do automation and AI play in DQM? Using ML to take over one or more DQM functions can enable a more consistent, continuous application of DQ policies across all data types. They reduce human intervention, accelerate responses, and boost productivity. Implementing them will make your entire DQM initiative more reliable, repeatable, and accurate.
Some specific examples of what capabilities can be automated by AI and metadata-driven automation are:
- Matching duplicate data.
- Proactively sense and remediate DQ issues.
- Suggest DQ rules that need to be applied to new data or apply those rules automatically.
- Detect and alert of data anomalies, such as deviations from expected mean, minimum, or maximum values or suspicious changes in the frequency distribution.
- Detect and alert of changes in schema and volume
- Data domain and sensitive data detection
All of that is great, but here is the best part:
Even though they are using AI-driven DQM automation now, successful enterprises are more likely to expand their use in the next 12 months and invest further in AI innovations. They are continually looking to do better.
Want to learn how to establish automated data quality processes by using metadata and AI? Read our whitepaper!
Success factor #2: Infrastructure
You need a deep-stack DQM functional infrastructure for better outcomes with data quality. “Deep-stack” means that your data quality platform needs to be adequate to address all of your DQ issues. You need DQM tools that avoid on-demand processes that must be constantly renewed and repeated.
Our survey reported several “challenges” related to DQM directly linked to inadequate infrastructure. Some of the top problems our respondents reported were:
- Scaling DQM
- Mitigating DQM vulnerabilities that come with self-service data analytics apps
- Applying DQM to new sources of semi-structured & unstructured data
- Enforcing consistent DQM across diverse landscapes
- Identifying sensitive data via quality metadata, cataloging, and lineage
In terms of DQM infrastructure, our respondents who reported DQM success were also more likely to be implementing the following modernizations:
- Closed-loop remediation. Accelerates response to DQM issues through real-time alerting and response mechanisms within the DQM stewardship workflow.
- DQM Observability. Supports continuous measurement of DQM metrics such as data completeness, consistency, and accuracy. Provides a unified view of data logged on systems throughout the DQM pipeline.
Data catalogs are getting the attention they deserve.
Ever since the launch of Ataccama ONE Gen 2, we have advocated for using a data catalog to automate data quality because using metadata for data quality automation is more than practical.
Data leaders are increasingly buying into this mindset because 45% of respondents say they are implementing a central data catalog in the next 12 months. Moreover, those who reported success were more likely to have catalog implementation in their plans.
Besides these modernizations, there are also industry-standard capabilities that every DQM initiative should be utilizing. You’ll find those below.
- Self-service DQ reporting, analysis, and dashboarding. Tools that allow business and technical personnel to configure, consume, analyze, and share data quality information. Our respondents also spoke highly of tools with task-focused UX, low code visuals, and high customizability.
- DQ Alerts. (Preferably AI-driven and automated) Alert individuals about DQ issues in a guided workflow to resolve them. AI contextual recommendations presented in a self-service dashboard are also a significant advantage. 29% of our respondents employed AI and automation for their DQ alerts.
- Collaborative Data Stewardship. An online environment with workflows, sharing, and other collaborative features brings users together for DQM functions.
- Central Rules Library. Author, revise, introspect, and supervise the business rules embedded (and enforced) by one or more DQM functional components. 39% of respondents have this.
- Issue Remediation. Dashboards and tools that have a collaborative review and remediation of DQ issues.
Success factor #3: Standardized deployment and commitment to DQ practices
Those respondents who identified as successful were more likely to have teams in place to address DQ, whether distributed in business units or enterprise-wide.
Consistency is pivotal for success with any DQM initiative. You need to think about two areas:
- Standardized deployment keeps the organization’s data quality policies, procedures, and structures consistent.
- A common mindset keeps everyone at your company on the same page about what’s expected of them and the company’s data system.
Standardized deployment
When it comes to deploying data quality processes, there are two paths you can take: centralized or decentralized. In the centralized approach (43% of respondents), you will have consistent and universal DQ tools and policies throughout the entire enterprise and a single data governance team. In the decentralized approach (51% of respondents), your data systems and policies will work on the business-unit levels, having global governance with a few open standards and individuals in each team responsible for their respective datasets.
Common mindset and data quality culture
An enterprise’s culture should prioritize maintaining high data quality for all business and technical roles. Our respondents listed “convincing employees to adhere to DQM policy” and “training employees in DQM compliance” as two of the biggest challenges with their initiatives. If employees aren’t up to speed with your company’s plans for DQM, you can guarantee they will slow down or inhibit the initiative.
To keep employees educated and morale high, you’ll need to focus on creating governance policies that:
- Are clear and usable
- Offer guidance to stakeholders
- Are workable in everyday operations
- Minimize the need for specialized DQM training
- Avoid the need for technical support when implementing
38% of our managers’ respondents also reported issues with receiving executive support for the DQM plans. This can greatly slow down the process as initiatives require much support at the C-level to ensure they are adequately funded and allotted the appropriate amount of time to perform effectively. Establishing steadfast top-to-bottom data quality support could begin with implementing an enterprise-wide coordinating council (as long as you keep it lean and agile).
Above all else, it’s essential to acknowledge that any DQ project is ongoing. It takes time to reach data quality maturity and will require much patience and receptiveness from all parties involved.
Bonus Information: Data Quality Maturity
You might wonder where you stand regarding data quality maturity compared to other organizations.
Below, you’ll find the data quality maturity matrix, including tell-tale signs to determine your organization’s maturity. Of course, you can find more details about each component in the full report.
Learn more about our data quality solutions to see what Ataccama ONE is capable of!