The Top 5 Initiatives CDOs Focus on to Bring Value in 2024
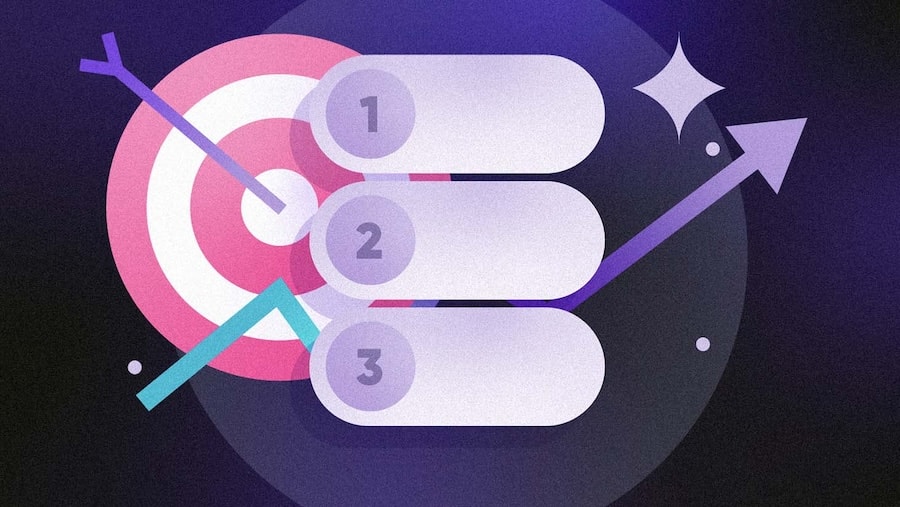
What is success?
If you’re a CDO, you might have debates about this with yourself and your peers on a regular basis. Data tells that 42% of CDOs define success in terms of achieving business objectives, while 33% see success as a combination of business and technical objectives.
Clearly, the role of the CDO is moving further away from technical wins and “data programs” and closer to “business value.” That means that whatever next great thing you bring to the organization must lead to business outcomes: new products and markets, employee productivity, customer value, and anything else that contributes to the top and bottom line—directly or indirectly.
So, do you want to be in the cohort of CDOs that bring business value? Get inspired by what others are doing!
Read on to learn about the top 5 key initiatives CDOs focus on in 2023, according to two latest CDOs studies.
#1 Data governance is not going anywhere
Data governance is one of the most value-generating activities for CDOs.
According to 2023 AWS Chief Data Officer (CDO) Report, “establishing clear and effective data governance” is the top reported responsibility for CDO (51% said it was on their list of responsibilities, and 66% said it was one of the activities where they spent 20% or more of their time).
These stats are not surprising because data governance goals are availability, trust in data, and data protection. No data strategy can be executed efficiently without a data governance component. In fact, the same study shows that data governance is the #1 avenue to value creation.
According to a study of CDOs in Japan, establishing a data governance program was the first objective on the CDOs’ list. After that, they focused on building and operationalizing a data platform for analytics and general utilization.
With its wide-reaching effects, data governance remains a vital (and perennial) area of focus for CDOs.
At what stage of data maturity should you focus on data governance?
Data governance is a broad discipline, so your focus will differ along the organizational maturity levels in its processes and data culture.
Depending on your organizational goals, data governance should deliver a mix of short-term wins and long-term foundations.
So, a more appropriate question is: what to focus on at different stages of your organization’s data maturity?
At lower maturity levels (you’re trying to establish standardized reporting):
- Centralize reference data management.
- Set up the most critical data quality checks for the data used in corporate reporting.
- Establish a business glossary to enable a shared understanding and use of data.
At intermediate levels (you’re trying to make data available throughout the organization):
- Make tools like the data catalog accessible to a wider audience.
- Document and make data policies available to all employees.
- Invest in data literacy training
- Make data lineage available to Data Engineers and other core data professionals
- Establish an MDM hub to build golden records for the key record domains in your organization.
- Set up AI-based data quality monitoring and data observability tools to expand and automate the coverage of DQ assurance.
At advanced levels (you’re trying to enable data-driven decisions at all levels of the organization):
- Make data-driven decision-making part of the corporate culture, embedded into meeting agendas, investment requests, corporate performance management, etc.
- Automate data access controls and PII protection.
- Preventing data quality issues by integrating DQ checks at various data entry portals and apps.
- Provide self-service tools for data quality management or data analysis.
#2 Data modernization might be what you need to get your data strategy to the next level
Data modernization is a relatively new concept, so definitions differ, but here’s our version:
Data modernization is the process of improving an organization’s data infrastructure and processes to improve the efficiency, reliability, accessibility, and usefulness of an organization’s data assets.
According to Data and Analytics Leadership Annual Executive Survey 2023, data modernization is the top investment area for CDOs in 2023, with 82% planning to increase spending throughout the year.
Data modernization involves the whole data stack:
- Storage
- Ingestion
- Transformation
- Monitoring & Governance
- Analytics
And it doesn’t just mean “moving to the cloud.”
It means, given the stage of your data maturity, your data strategy, and the goals on it, optimizing the data stack to do one or all of the following:
- Reduce costs and TCO for the data stack.
- Improve the speed of data processing and provisioning.
- Enable things you can’t (but want and need) do right now; for example, moving to an operational model of launching and running data products.
When should you focus on data modernization?
Data modernization can be relevant for companies at different levels of data maturity. For example, companies just starting out with the strategic use of data & analytics might find it necessary to modernize their data stack after several successful analytics projects because they want to scale them, deliver them faster in the future, or use more data.
Conversely, companies that were early adopters of data management technology might find it necessary to update to cloud-based alternatives and tools with better deployment and integration options.
The decision to modernize the data stack usually needs to be justified by a solid ROI, but the drivers usually are:
- Increasing costs of data management
- Poor user experience
- Your needs begin to outgrow your current vendors’ capabilities
- Growing complexity in the data stack, breaking integrations, and increasing costs to maintain them
- Highly manual processes to find, access, and prepare data
In the end, data modernization is a highly individual activity (and likely a heavy lift), which involves many stakeholders in the company, with a slow, gradual realization of value.
#3 Data products are the hottest data trend
Data products are still a very new concept with varying definitions.
The idea comes from the concept of the data mesh and one of its pillars: data as a product. Once applied to data, you can produce data products with the following characteristics:
- Sharable and discoverable: publish data products for the rest of the organization to use.
- Self-describing: data products should be very well defined.
- Addressable (same location, predictable in the future): data products should be able to be accessed.
- Trustworthy: data products should have high data quality.
- Interoperable: data products should work with other data products.
- Secure: protected from unauthorized access.
According to Eric Weber, “In a data product, your data is the key differentiator that enables your competitive advantage.” It happens in a couple of ways:
- Data in your product enables a unique user experience
- Data in your product is not readily available outside your organization
- Brings business value
Examples of data products are:
- Raw, integrated data
- Aggregated data
- Dashboards
- ML models (the model itself is a differentiator that relies on the data that you have and provides a unique end-user experience)
- Experimentation platforms
What makes data products data products (and not just data assets) are the characteristics we mentioned above and the product management thinking that goes along with building data products.
At what stage of data maturity should you focus on data products?
Adopting a data products mindset requires quite a high level of data maturity.
That’s why the concept has been primarily adopted by large companies with large data teams, modern infrastructure, and advanced analytics capabilities. For these companies, adopting the data-as-a-product mindset simplifies access to insights and speeds up business initiatives that depend on data.
If you review the characteristics of data products, it becomes clear that achieving them requires solid data governance foundations and a mature data stack covering data quality, metadata management, data lineage, ETL, and data warehousing/lake, at the very least. Some thought leaders have even started talking about combining the data fabric and data mesh approaches to deliver data products.
To conclude, focus on building data products if you have the components necessary to produce consistent reporting and support analytics projects.
#4 AI & analytics is a tried & true method to bring value
CDOs worldwide see analytics & AI as one of the top ways to bring value to their organizations.
According to 2023 AWS Chief Data Officer (CDO) Report, 36% of CDOs “focus on a small set of key analytics or AI projects” to bring value, and that’s not surprising. Studies show that companies are getting ROI on their data, and they don’t need a lot of models in production to do that. A 2021 McKinsey survey unveils that the share of respondents reporting at least 5% of earnings (EBIT) attributable to AI has increased to 27%.
In 2023, AI initiatives remain high on the list of CDO priorities, with 80% reporting increased investments in this area. Let’s not forget about Gen AI, which has taken the world by storm: executives are evaluating how to apply it to increase productivity while software vendors are incorporating LLMs into their products.
At what stage of data maturity should you focus on analytics & AI?
At the beginning of their data maturity, companies should focus on supporting analytics initiatives with high-quality data, even in regulated industries where data defense should be given a lot of attention.
Investing in ML capabilities (similar to developing data products) requires:
- Data governance foundations
- Tools for easy data discovery like the data catalog and processing to ensure data quality
- Robust processes around the building, reviewing, deploying, testing, and monitoring AI models.
#5 Data quality is still in high demand
Data quality is foundational to every data-dependent activity.
While not a new cool kid on the block, data quality management underpins anything from the basics, like accurate reporting, to advanced undertakings, like creating data products and building ML models. It’s a simple but effective method that you can quickly get started with for one particular project and then expand to others until you make data quality management an enterprise-wide program. In fact, some organizations focus on data quality, even without launching a data governance program.
It’s not shocking then that CDOs find data quality as one of the top areas of focus: 78% state that “improving data quality” is one of the responsibilities included in their job. It’s worth noting that only 50% of organizations successfully manage data quality. Learn more about the state of data quality by reading our original research.
At what stage of data maturity should you focus on data quality?
It might be clear now that data quality does not require any organizational data maturity—just acknowledging that you have a problem with data quality. If you are a data-driven organization, focusing on data quality in at least its rudimentary forms is a must.
2023 CDO Focus Mix
With everything said, here is an extra table summarizing the required data maturity, effort, and time to outcome for each investment area that we covered in this article.
Area of investment | Required data maturity | Effort | Time to outcome |
Data governance | Low | Medium to high | Slow to medium |
Data modernization | Low to medium | Medium to high | Slow to medium |
Data products | Medium to high | Medium | Medium to fast |
AI & analytics | Medium | Medium | Medium |
Data quality | Low | Medium | Fast |
Looking to bring value to your organization?
We provide a unified, modular data management platform that serves as a backbone for all of your data initiatives.