Explore your company’s data
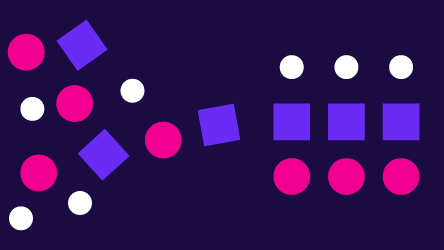
Handling massive amounts of data can be challenging. Scrolling through lengthy columns and volumes might slow you down, cause you to miss out on opportunities, or even lose sight of the value you have at your disposal.
That’s why companies need a tool for sorting that giant pile of records to get the information – and the value – they are searching for. Enter the data catalog, a comprehensive data management solution aimed at sorting, tracking, and searching for data.
This blog teaches about data catalogs, why you need them, what to look for in a data catalog solution, and much more. Let’s get started.
Introducing the data catalog
Discover, understand, and leverage your organization’s data assets like never before. A data catalog is an all-inclusive solution that empowers your business to efficiently organize, search, and utilize data across the enterprise.
Use the data catalog to upload all your data sets, explore your data, and instantly access the records your company relies on for informed decision-making – further unlocking the full potential of your data ecosystem.
An adept data catalog can make the lives of data scientists, engineers, and business users more accessible by providing them with streamlined access to the records they need for their daily and long-term responsibilities.
Why do you need a data catalog?
A data catalog is an essential tool for any data-driven organization. It comes down to four key pillars that are vital to successful data and business practices:
- Collaboration. Data catalogs allow users to create and improve data assets collaboratively with features such as task workflows, commenting, and sharing.
- Governance. Implement your business and data governance into the catalog to synchronize data and business regulation, providing faster and more secure access to data.
- Automation. Automation is critical in any industry, especially in today’s data landscape. Keep your systems constantly up to date with automated data discovery and observability.
- Activation. Now, your data catalog is ready to go. With greater access to data, you can use it to create metrics, reports, and trusted data sets and export that information internally and externally.
Key features and benefits of data catalogs
Now that we’ve established the importance of data catalogs, let’s cover the essential features you should consider when searching for a solution.
Data ingestion and discovery
Once you choose a catalog solution, you’ll need to connect it to all relevant data systems in your company. Your catalog should have pre-built adapters to discover metadata and make the ingestion of data automatic. Also, it will need a mechanism for data discovery, which should be ongoing as new data sets appear.
Search
A data catalog is basically the “Google” for your company’s data systems, providing a search engine for finding the information you need. That’s why a “search” functionality is essential: people can discover the data they’re looking for in a timely fashion. AI suggestions in search can make this function even more effective.
Business glossary
This is the catalog section where you can store business terms to help end users understand the meaning, origin, and recommended use of the data they’re working with. The business glossary should also be integrated with external applications to keep the definitions of these terms consistent.
Metadata management
Good data catalogs allow you to freely add additional metadata and tag your terms with things like a data category (e.g., sensitive, GDPR, PII related, track business owners) and any other important information. Even more advanced catalogs will employ AI to automatically tag assets with metadata, making them easier to locate and organize.
Data lineage
Data lineage helps users understand the origin and destination of any data asset in a data catalog, how the data was transformed or enriched on the way to obtaining the final result, and how different pieces of data are related. It helps comply with regulatory requirements and ensures the proper treatment of data throughout any pipeline stage.
Data quality monitoring and anomaly detection
Your catalog should also allow you to keep a pulse on the quality of your data. Constantly reviewing the quality of incoming and existing data via data quality monitoring and using AI-powered anomaly detection to spot inconsistencies invisible to the human eye will give your solution a great advantage.
How a data catalog works
You might think, “All of this sounds great, but how do I use a data catalog?” We’ve outlined the following scenario to illustrate how a user might interact with the catalog daily.
Step 1: Logging in
Our User, Danny Data, starts their day by logging into the data catalog platform using their credentials.
Step 2: Begin browsing data assets
Once logged in, Danny navigates to the data catalog’s homepage or dashboard. Here, they see a list of available data assets, including datasets, databases, tables, files, reports, etc.
Step 3: Search for specific data
Danny has data analysis tasks for a specific data set, so they use the search bar or filters to find the particular data they need. They might search by keywords, tags, data types, or categories.
Step 4: Exploring metadata
Upon finding a relevant data asset, Danny clicks on it to explore its metadata. Metadata includes information about the data, such as its source, description, format, owner, creation date, quality, and relevant tags or labels.
Step 5: Assessing data quality
Before Danny can use the data for analysis or reporting, they check the data quality metrics provided in the catalog so they don’t use unprepared or unsatisfactory data for their work.
Step 6: Viewing data lineage
Danny is very thorough with his work, so he wants to understand the data’s origins, transformations, and dependencies before using it for critical decision-making. This helps ensure data integrity, traceability, and regulatory compliance.
Step 7: Accessing data
Once Danny is satisfied with the data quality and lineage, he can access the data directly from the catalog. Depending on permissions and access rights, they may download the data, connect to it via APIs, or preview it within the catalog interface.
Step 8: Collaborating and sharing
Danny collaborates with the rest of his data analysis team by sharing links to the data assets or adding comments and annotations within the catalog. If they have questions or follow-up tasks, they might request access to additional datasets or provide feedback on existing ones.
Step 9: Logging out
At the end of the day, Danny logs out of the data catalog platform to ensure data security and privacy.
Use cases and success stories
As a data management company, we’ve been privileged to help several businesses build, maintain, and use their data catalogs. Check out some of our greater success stories below:
Teranet
Catherine Yoshida was hired to establish a data governance practice in their company to expedite the data product-building process. She started with a data catalog and governance because they are the basis for any data management activities. Without it, you can’t do much else. Then, she plans to expand it to DQ and, lastly MDM (learn more).
T-Mobile
T-Mobile needed to identify sensitive and PII data to avoid breaches and, thus, regulatory penalties. They needed to be able to scan their large amounts of data reliably and at scale, classify them, and uncover the blind spots. To solve this, we helped them implement an automated catalog with DQ on top of it (learn more).
Getting started with a data catalog
Need some more confidence before you get started? Here are some tips about getting started from one of our data catalog experts:
- Identify the use case and business needs. For any data program to be successful, it needs to have a buy-in from execs. Understanding what you want to achieve will also impact what catalog you choose. There are different solutions on the market, and they all have slightly different capabilities. So, understanding your goal will help you make the right choice.
- Connect it to all your data sources and surface data. Run data discovery and profile your data to gain deeper insights into your data sources: analyze patterns in data, understand which business terms have been assigned or which DQ rules have been applied, and if any anomalies have been spotted, etc.
- Create a glossary of business terms. Make sure you collaborate with business people on the term descriptions to create alignment across the organization. This way, anyone using data from the catalog will understand the asset and how to use it.
- Make sure you have the governance structures in place. This includes stewardship (i.e., who has access to what, how they can use it, who owns which data asset) and the ownership hierarchy (so if something happens, people know who to contact to perform necessary actions on the data set), etc. Basically, define a set of permissions.
Let Ataccama help you set up your data catalog
Don’t just rely on crowdsourcing. Automation keeps a catalog up-to-date and is a must for the long-term survival of your data governance initiatives. Any tool you select for the job should help you achieve this. If this sounds great, learn more about our automated data catalog here.