Boost Data Catalog Crowdsourcing with Automated Metadata Capture
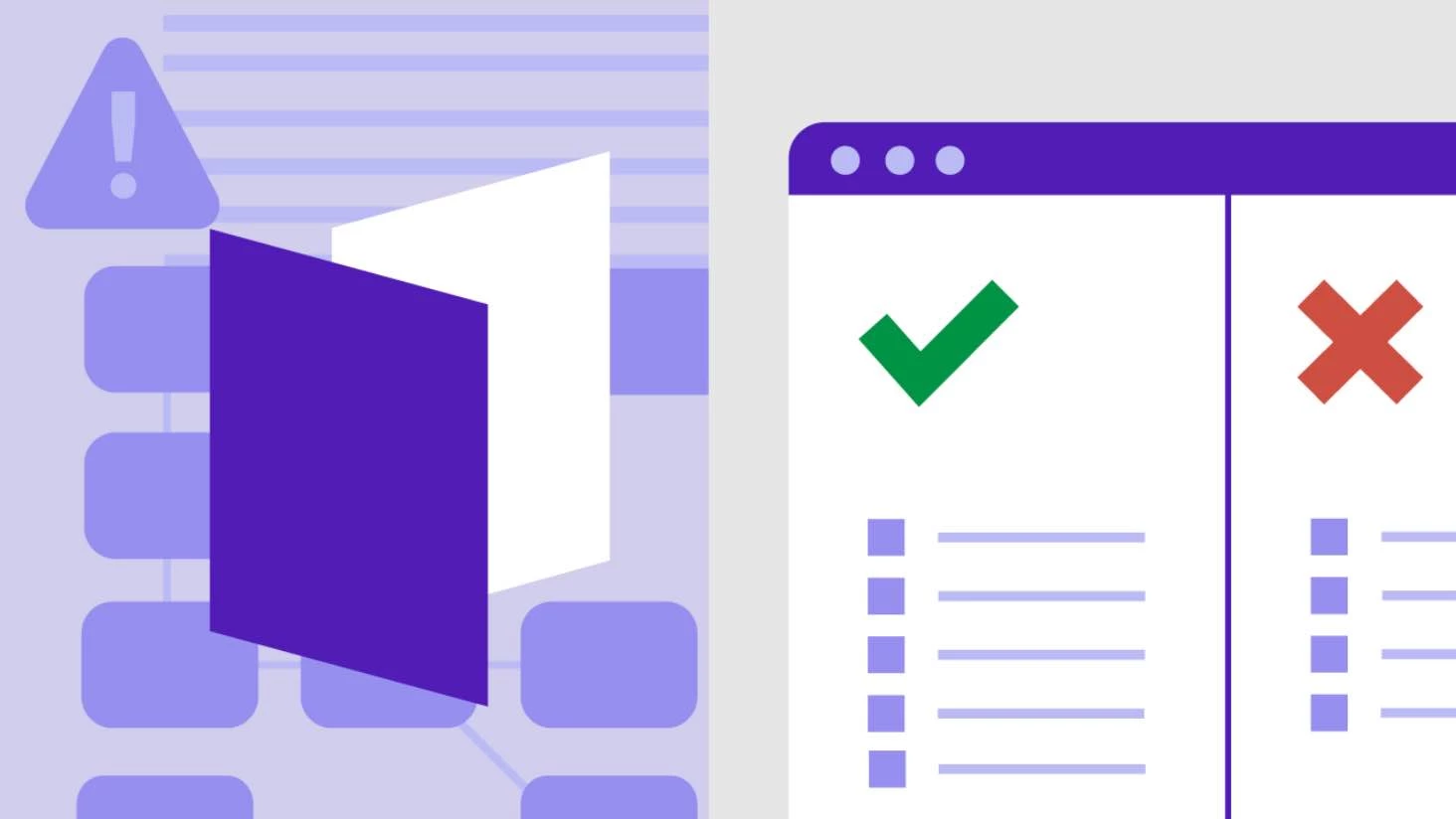
When you hear the words “data catalog,” you’re likely in for some opinions about the importance of crowdsourcing in generating business metadata. In fact, some people argue that crowdsourcing is the feature that makes data catalogs (and metadata management) so powerful and effective.
It’s no doubt true that crowdsourcing is a great data catalog capability. After all, it enables teams and departments to make their tribal knowledge of particular data available to everyone. However, crowdsourcing is more efficient as a second step, after a catalog has been populated and enriched with as much business metadata as possible.
With that in mind, what businesses really need is to automate the generation of business metadata. Why? Because the traditional process of populating a data catalog, which relies on crowdsourcing business metadata, is long and tedious. It’s worth a closer look.
The Challenging Task of Crowdsourcing Metadata
Organizations that want to introduce a data catalog generally assign the task of populating it to a small team of data stewards, who are then responsible for launching a ready-to-use solution for the rest of the organization or department. As anyone who has tried it will know, this is far easier said than done.
Most data catalog solutions connect to data sources and import technical metadata. What that usually means is thousands of tables and columns named something like XDS_E2121_A_32. These naming conventions don’t make the task easy for the data stewards. While end users and data owners sometimes possess the knowledge of what is really inside those data sets, often they are reluctant to create and maintain metadata for those data sets.
At the same time, existing metadata documentation in the form of, say, Excel spreadsheets is often not easily available. In other cases, it is outdated, incomplete, or inconsistent with other departments’ documentation for the same data source. Database information schemas are no better: they are unlikely to have been updated since the day they were created.
Therefore, it is up to the data stewards to make inquiries and populate the catalog with relevant, up-to-date business metadata, such as business domains, classifications, and data quality indicators. A task such as this takes anywhere from months to years to complete. Effectively, data stewards face what writers call “the fear of the blank page.” Where to start, and how to get from 0 to 1?
Even if we imagine that over the course of a year of painstaking manual population, the data catalog is finally “complete,” let’s not forget about the crucial element of keeping metadata up to date. After all, data is dynamic: schemas change, data comes and goes, human error is, to some extent, unavoidable. How, then, to keep up with the pace of change in data and metadata?
If crowdsourcing alone were the answer, it would require an unprecedented data culture, processes, and accountability. It’s clear that this is not the case in the world today—and perhaps not ever.
So, is there a better, simpler way to introduce a data catalog solution into an organization and keep it useful afterward? Fortunately, yes. The answer lies in a new realm that will soon revolutionize data cataloging: automation.
A Better Way: Automated Metadata Capture
Substantial portions of the metadata capture and catalog population can be performed by the catalog solution itself. Let’s look at some examples:
- Data domain discovery: Detecting Personally Identifiable Information (PII) domains (names, addresses, emails, gender) or organization-specific domains (such as product or customer types).
- Data profiling: Automatically generating a data profile for every data set imported to the data catalog.
- Data quality evaluation: Evaluating data quality based on a detected domain.
- Reference data detection: Identifying reference data by analyzing the contents of a column in a data set.
- Lineage: Automatically generating data lineage, enriched with business metadata, for each data set when it is imported to the data catalog.
- Anomaly detection: Detecting irregularities and changes in data quality and characteristics.
- Detecting changes in metadata: Detecting changes in dataset structures: new and deleted columns, changes in column names and data types, significant changes in contents of data, and changes in classifications (e.g., detection of new PII data).
- Relationship discovery: Detecting foreign key candidates between two data sets by analyzing data.
- Policy enforcement: Applying data governance policies across data sets automatically, based on available metadata.
Immediate Insights for Data Stewards
So, how would automating these tasks help data stewards create an information-rich data catalog?
Thanks to the automatically captured metadata, they will immediately know:
- Which systems have the worst data quality.
- Which systems are used the most (thanks to lineage).
- Which systems contain data with the domains of interest (for example, regulatory-driven PII).
With pre-populated metadata, generated data profiles, and calculated data quality, data stewards can now focus on analytical activities, rather than documenting metadata and manually identifying problems in their data, one system at a time.
This information gives data stewards a great starting point, from which they can jump into the real substance of the work and deliver results faster.
Now that the stewards understand the state of their data, they can:
- Pass on their findings to data governance specialists to create and improve policies.
- Collaborate with data owners by identifying deficiencies in data in particular source systems and suggesting ways for improvement.
- Present their findings to the CDO and prioritize which systems need improvement.
Clearly, automating metadata capture and catalog population is a more efficient way to reap the benefits of a data catalog solution. By extension, maintaining metadata becomes much easier, too.
Crowdsourcing as a Control Mechanism
Of course, we are not going to discard crowdsourcing altogether. In fact, here is the moment for it to shine. After data stewards and other actors begin implementing changes to improve data governance and data quality in a particular system or set of systems, they can involve data experts and the frequent data users of given systems. Together, they can add additional metadata, validate the results of the automated processes, and make improvements manually. The “crowd” thus acts as a control mechanism for the automated processes.
As a result, collaboration starts around a specific problem or area for improvement. For example, data experts can identify the root cause of data quality problems in a particular system. Also, they can review the results of data domain detection (business term mapping) and identify data attributes or data sets that were not tagged. Together with the business term owner, they will alter the detection mechanism accordingly to make sure this and similar data attributes are tagged with that business term in the future.
In this way, step by step, teams remediate data quality issues, improve metadata of individual items, and make automated processes more precise.
Metadata Management Automation for the Enterprise
By now it should be clear why relying solely on crowdsourcing for populating a data catalog is not a safe bet and why it works best as a second step. But you might still be wondering: what exactly does metadata management automation bring to the enterprise? Below is a summary of the most impactful benefits:
- Massive reduction of the time to value of the initial implementation
- Increased involvement of the various stakeholders with crowdsourcing by providing a starting point around which to base the discussion, collaboration, and improvement
- Greater ability of organizations to efficiently scale and catalog an unlimited volume of data from multiple sources
- Significant reduction in the time needed for metadata maintenance
These new automated metadata management capabilities and their benefits present a big opportunity for enterprises to streamline data governance, operationalize metadata, and kickstart data management.We have builtAtaccama ONEspecifically for this purpose. It is a self-driving data management platform with AI and automation at its core. With continuous data discovery and data catalog at the center, Ataccama ONE provides active metadata to power and automate data quality, master data management, and data governance.