The dynamic duo of data and generative AI
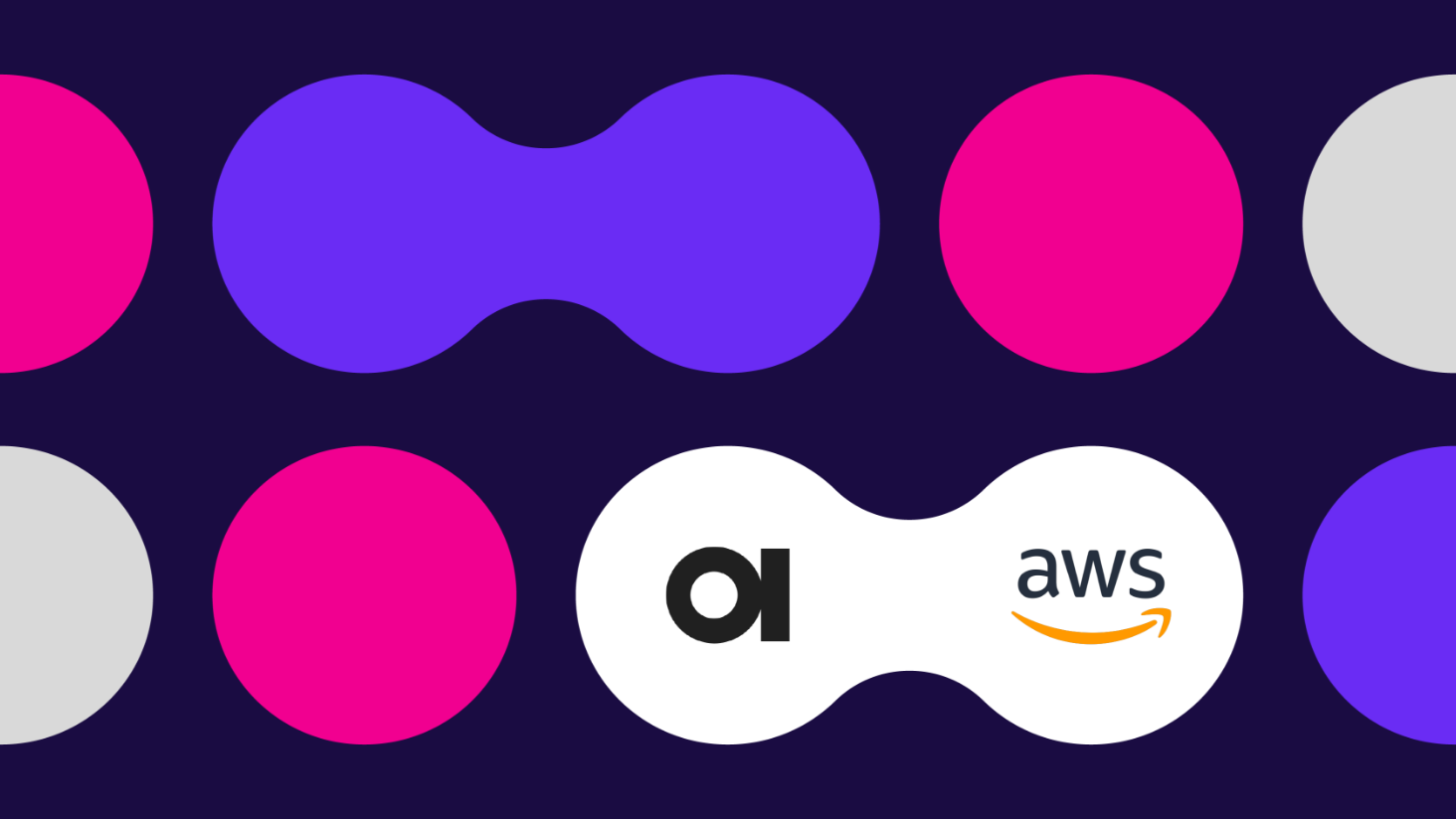
Written By Ahmed Salah: Ahmed Salah is Sr. Partner Solutions Architect at AWS. He’s focusing on AI/ML & Customer Experience CX. In his role, his mission is to help organizations solve intricate business problems, drive successful outcomes, and enhance customer experience.
Imagine a world where your data becomes not just a valuable asset but a dynamic force driving your organization toward greater innovation and success. This vision is no longer a distant dream but a reality today.
Generative AI can transform the data landscape, with Goldman Sachs forecasting a 7% (or almost $7 trillion) increase in global GDP and a 1.5% productivity lift over ten years. About 300 million jobs are likely to be impacted by AI.
Generative AI, at its core, rides the wave of the latest in machine learning. With over two decades in the artificial intelligence and machine learning AI/ML arena, Amazon and Amazon Web Services (AWS) have democratized these technologies for customers to leverage and derive value from – a true reflection of Amazon’s heritage, ethos, and future vision.
Throughout this blog post, I’ll delve into data’s pivotal role as a differentiator in building generative AI applications. I’ll also share key considerations that organizations should consider to effectively harness the power of generative AI, highlighting how strategic data management lays the foundation for success in driving transformative business outcomes.
Navigating the interplay of data management and generative AI
Embarking on the journey of building groundbreaking generative AI applications, we quickly discover that the true differentiator lies within the DNA of an organization – its data. In the vast landscape of foundational models, the companies destined for success harness the power of their unique datasets.
For instance, consider an online travel agency aiming to curate personalized travel itineraries using artificial intelligence. The secret sauce lies in utilizing customer profile data to tailor recommendations based on past trips, web history, and travel preferences. This invaluable data synergy extends to incorporating additional company data, such as flight and hotel inventory, promotions, and other travel intricacies.
As the cornerstone of digital transformation, data fuels insights that propel organizations forward, influencing every application, process, and business decision. It is the rocket fuel for digital transformations, supporting emerging use cases like generative AI and steering businesses toward scalability and profitability.
Yet, a significant challenge persists – organizations possess vast amounts of data but struggle to extract its total value. A survey by McKinsey reveals that only 25 percent of B2B companies use data weekly to understand customer needs, while 9 percent never utilize data at all. The central question is clear: How can organizations harness their data to achieve transformative outcomes?
How to use data management to maximize the potential of AI initiatives
As the volume of data grows, businesses seek smarter decision-making and a deeper understanding of their customers. Below, I will outline strategies for leveraging the right people, processes, and technology to extract maximum value from your data and drive a successful digital transformation.
Data proliferation
Efficient integration across varied data streams is pivotal for effective data management strategies. Emphasizing robust cataloging and governance tools can lay a solid foundation for comprehensive data lakes.
Consider leveraging purpose-built storage solutions, data transformation tools, and access management protocols to ensure secure and efficient handling of diverse data types.
Additionally, integrating generative AI applications can benefit from utilizing various database technologies tailored to store contextual information and facilitate personalized responses. Exploring vector databases for similarity and semantic search can further enhance storage and retrieval processes crucial for supporting generative AI applications.
Data integration
Merging data from various sources and formats into a unified, usable format is often laborious, demanding extensive data transformation and integration efforts. Adopting solutions that prioritize seamless integration, scalability, and security is imperative in data-driven architectures.
Utilizing a robust data lake strategy can break down traditional data silos, enabling agility and scalability in analytics. By employing tools like automated data extraction, cleansing, and consolidation, organizations can streamline their data workflows without the need for extensive Extract Transform Load (ETL) processes. Features such as centralized data catalogs, automated data preparation, and built-in security measures further enhance the efficiency and reliability of data operations.
Data quality
Maintaining data accuracy, completeness, and consistency is a constant battle. Inaccurate or inconsistent data can lead to flawed decisions and operational inefficiencies. By implementing adept data quality measures, engineers can ensure the reliability and accuracy of their datasets, thereby enhancing overall data confidence.
For instance, automated rules tailored to specific datasets can help maintain freshness and accuracy. Tools like Ataccama, with their Gen AI-powered rule suggestion, can help with this. By integrating such rules into data pipelines, organizations can prevent poor-quality data from infiltrating their data stores and promptly identify any degradation in data quality. This proactive approach to data quality management empowers organizations to build applications and insights on a solid data foundation.
Data access and usability
Offering secure and user-friendly data access in a format that suits the end-users needs is an ongoing challenge. Organizations want to gain insights into their data to make informed decisions. While modern business intelligence (BI) has significantly expedited workflows and simplified data utilization, we still face a challenge in achieving widespread, continuous, data-driven decision-making.
A recent survey by Gartner highlighted the major obstacle to success in data & analytics (D&A) – a shortage of data skills and staff. Analysts dedicate a substantial portion of their time to intricate, multi-step tasks, often involving learning new tools and proprietary languages. Simultaneously, business users grapple with a lack of knowledge and skills required to extract value from their data. Generative BI authoring can empower business analysts to create and customize visuals effortlessly using natural language commands to address this data access and usability challenge, offering solutions that enable analysts and business users to derive meaningful insights.
Also, by leveraging generative AI-powered digital assistants, organizations can streamline tasks across functional groups, fostering efficient workflows and informed decision-making.
Data governance
Navigating the intricate landscape of data governance in the generative AI domain poses unique challenges. The struggle to balance access and control becomes particularly pronounced, with the risk of stifling creativity through excessive restrictions or compromising security with too much access.
Generative AI introduces additional complexities, demanding meticulous data quality, integrity, toxicity, and bias governance. Businesses can streamline data management processes and expedite informed decision-making by implementing comprehensive data governance strategies.
One practical approach involves deploying a centralized data hub solution to facilitate seamless data curation, discovery, and protection across disparate data sources. Such an approach empowers teams to catalog, access, and govern data efficiently, regardless of location or origin.
Building a strong foundation for responsible innovation
With a strong data foundation, navigating the landscape of generative AI applications becomes smoother. As you embark on this journey, it’s crucial to consider a range of foundation models that can responsibly support your organization’s needs at every adoption stage. Ensuring enterprise-grade security and access to leading models are paramount to accelerating innovation and deriving business value.
In tackling various business problems, it’s essential to understand that no single model fits all scenarios, and constant model improvements necessitate stitching together different models and versions while maintaining data security and privacy. This can be resource-intensive and time-consuming.
Consider leveraging fully managed services, which can provide access to high-performing models. Simplify experimentation and customization using techniques like fine-tuning and retrieval-augmented generation (RAG) without delving into coding complexities.
Furthermore, consider employing autonomous agents or frameworks within your application to orchestrate and streamline user interactions with organization data, APIs, and knowledge bases for executing intricate business tasks. Also, implementing tailored safeguards aligned with your specific application needs and ethical AI guidelines is crucial.
Conclusion
The integration of generative AI has emerged as a paramount focus for executive leadership within organizations, with Gartner predicting that more than 80% of enterprises will have used generative AI APIs and models and/or deployed generative AI-enabled applications in production environments, up from less than 5% in early 2023.
Navigating this landscape effectively requires a concerted effort toward organizational transformation and addressing specific business challenges. To this end, executives must lead top-down initiatives that recognize the strategic importance of data to foster a culture of data-driven decision-making and innovation.
Additionally, it’s essential to encourage executives to identify and articulate their business challenges, enabling targeted application of generative AI to drive immediate improvements in customer experiences and business outcomes. By embracing comprehensive data strategies and integrating generative AI solutions, organizations can unlock new insights, foster innovation, and gain competitive advantages.
As executives embark on this transformative journey, leveraging resources like the Generative AI for Executives course on AWS Skill Builder can further deepen their understanding and maximize the potential of generative AI for organizational success.
We always appreciate collaborating with experts like Ahmed! Visit our AI solutions page to learn about our offerings!