What is Data Quality Monitoring
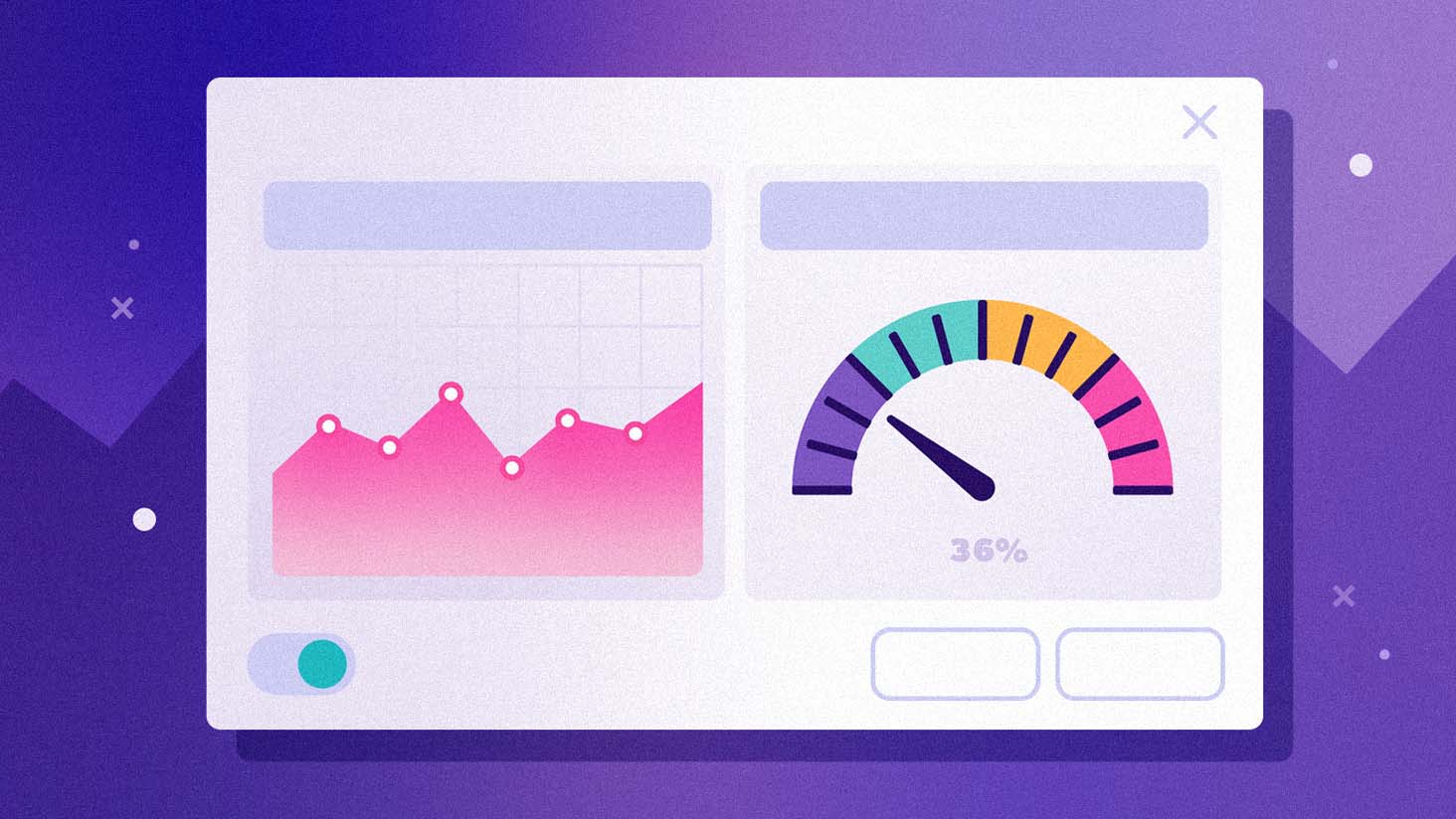
In the data world, every decision and strategy leans heavily on the information we gather. Whether it’s a marketing campaign or a new IT initiative, the quality of the data you’re using will directly impact the project’s success. That’s why keeping a finger on the quality of your data is essential to any business.
What’s the best way to keep track of data quality? Data quality monitoring, of course!
What is data quality monitoring?
Once all of your data quality systems are in place, you must consistently assess your data’s quality to ensure it meets standards and fits its intended use. This is achieved by a process called data monitoring.
Using the criteria set by your business rules, data quality monitoring tools will continuously check incoming and existing data (through data profiling or monitoring tools) to spot any deficiencies.
You can monitor data quality as often as possible but keep your intervals consistent. After you run monitoring, you receive information about all the dimensions of data quality:
- Accuracy
- Completeness
- Timeliness
- Validity
- Uniqueness
- Accessibility
In some cases, data quality monitoring can also deliver a tangible “data quality score” based on the findings from your DQ dimensions.
Why is data quality monitoring important?
Data quality monitoring helps you understand whether you can use/trust your data for decision-making and other business functions/initiatives. For data-driven companies, it’s just essential.
Whether you’re at the source level looking for null values in columns of data, improving analytics by finding formatting issues in customers’ date of birth, or validating addresses for email campaigns, monitoring data will give you the information you need to make these processes effective.
Companies that DON’T monitor their data will immediately see a decline in their data quality, leading to several business costs and risks, such as:
- Failed analytics projects
- Data that is non-compliant with government and industry regulations
- Hindered IT modernization projects
- Poor customer experience
- Employee dissatisfaction
Types of data quality monitoring
To understand how data quality monitoring works, we have to look at the different types of monitoring and their various use cases.
1. Metadata-driven AI-augmented monitoring
Metadata-driven AI-augmented monitoring provides a high-level DQ overview of every data asset in your catalog. It gives a surface-level understanding and trust in your data. It upgrades the catalog experience by providing additional information and data quality dimensions for all existing and incoming data. It’s great for data analysts, data scientists, or even business users because they can check whether a data set fulfills their requirements directly in the data catalog.
It works by:
- Creating DQ rules and adding them to your rules library.
- Assigning rules to various business and data domains (i.e., address validation rules for customer data).
- AI automatically recognizes similar datasets and tags them with appropriate domains/labels.
- DQ rules are automatically applied to all existing and incoming data.
- DQ metadata for all relevant DQ dimensions provided in the catalog (i.e., validity, timeliness, completeness, etc.)
2. Precise/Targeted DQ Monitoring & Reporting
Precise/targeted DQM, or DQ reporting, runs data quality monitoring tasks on especially critical data warehouse tables or assets in the data lake. Using the same rule library as automated monitoring, you can run monitoring tasks on specific attributes or columns of data instead of checking entire business terms/domains.
This makes it more precise and allows you to deliver DQ results for whatever aggregation of data (collection or sets of tables) you need (i.e., instead of only checking an entire set performs at regular monthly intervals, you could use precise monitoring to check how a particular subset performs over two weeks).
This is useful for regulatory reporting because you can apply rules from the rule library that aren’t mapped to business terms. For example, if a new regulation comes out about retention periods for PII data, applying that rule to each business term could be a much more intensive project than automatically applying directly to the attributes.
It works by:
- Monitor specific tables/columns by applying rules to them
- Closely monitoring trends in different DQ dimensions (whether DQ is falling or rising)
- Proactively fix issues that are detected.
3. Pure AI
AI-powered data quality monitoring works by discovering patterns and inconsistencies (anomaly detection) in your data. Once it finds commonalities within data domains, it can run incoming and existing data against those standards, recognizing when unexpected changes occur. It will flag these values as “anomalies” and use your input to learn and improve.
AI monitoring is excellent for discovering “silent issues” or unknown unknowns in your data. If quality issues occur that you weren’t expecting, AI monitoring can recognize them and reveal them to you. You can be notified about inconsistencies and changes in the characteristics of your data so you can prevent unexpected problems from causing actual harm. This is a common feature of most data observability platforms.
It works by:
- Continuously scanning all datasets and looking for irregularities such as data volume changes, data load changes, etc. .
- Creating alerts when unusual entries/values occur.
What are the best practices for data quality monitoring?
Now that we understand what data quality monitoring is, why it’s essential, and its different varieties, let’s get into some best practices so your organization can implement them effectively.
1. Set a clear goal
DQ monitoring can have different goals for business and technical users. Know what you want to achieve with DQ monitoring, and then you can better decide which of the above methods best suits your use case (or which you should implement first).
- The first method is for gaining an understanding and trust in data
- The second is for specific requirements, such as regulatory reporting
- The third is good for tracking structural changes in data.
The first suits the goals of a business user, while another might have greater advantages to technical professionals.
2. Use all three types
To fully cover the data quality of all your systems, you’ll need all three of the monitoring types we listed above. This allows you to monitor for signs of bad data qualityat all levels of your data landscape.
You’ll need precise monitoring to handle more specific tasks and aggregations of data. Meta-data-driven Automated DQM can save time and effort, and pure AI/anomaly detection can help spot DQ issues you didn’t expect/weren’t aware of.
3. Assess the success of your DQM
Monitoring the changes in metrics like the number of monitored data sources, the time it takes to uncover an issue, the number of projects delivered with a DQ platform, and time reductions in getting ready data will also shed some insight into the success of your DQM initiative. Take feedback from both business and technical users to see what’s working and what isn’t.
4. Monitor the right metrics
Depending on your data quality monitoring tool, you might not be tracking all the necessary metrics to keep a pulse on your data quality. Remember to keep track of the seven most important data quality metrics:
- Accuracy
- Completeness
- Consistency
- Timeliness
- Validity
- Uniqueness
- Relevance
Work smarter (not harder) with data quality monitoring tools
By continuously assessing the quality of incoming and existing data, you protect your organization from costly data mistakes and progress-stopping bottlenecks. You’re providing a safe space where your company can produce reliable data and quickly get it into the hands of people who need it.
At Ataccama, we have a data quality monitoring tool that covers all three of the types we mentioned above. All our data quality monitoring tools are in one platform, so you don’t need to waste time switching between tools to get all the results of your monitoring initiatives.
Learn more about our data quality software or see it in action below by requesting a demo!